This opportunity is a portfolio of hardware and software techniques that seamlessly accelerate AI/ML applications, while saving on-chip and off-chip memory, bandwidth, and processing power.
These compute and compression methods have been shown to deliver a 3x boost in processing speed with 2x-4x increase in storage capabilities (up to 10x for natural language processing).
By exploiting ineffectual computations, weight sparsity, precision variability, and bit content, the accelerators transparently reduce the amount of work that needs to be performed by neural networks. The methods lead to the design of performance-, energy-, and/or cost-optimized computing engines for various application domains.
OPPORTUNITY
AI and Machine Learning (ML) applications are notoriously hard to develop and require hardware capable of supporting their rapidly increasing data processing, storage, and energy demands. Conventional computing hardware suffers from sluggishness, low-fidelity, inaccuracy, high power, and long uptime. These limitations are especially noticeable in energy-constrained edge devices for next-generation AI applications like computer vision, natural language processing, and sensing. The AI/ML hardware market was valued at $6.6B USD in 2018, and is expected to reach $91B by 2025, a 45.2% CAGR from 2019 to 2025.
Unlike other approaches, including new ASIC designs and software acceleration, these techniques capitalize on the expected behavior of AI and ML applications, particularly in the value-access streams. By targeting optimizations at the middleware and silicon hardware levels with pre-built models and designs, these accelerators require no intervention from machine learning experts. The technologies are ubiquitous and flexible to accelerate any application powered by a silicon chip.
STATUS
- Seeking collaboration opportunities with end users
- Patent: System and method for memory compression for deep learning networks
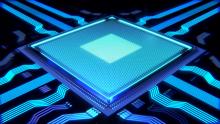